Decoding Healthcare Analytics: Definition, Types, and Importance
Table of Contents
Data is like a hidden treasure in today’s world. It’s most valuable when it’s cleaned up and made ready to use, kind of like how raw materials are turned into useful products. Data analytics is the process that changes simple data into something really valuable.
This change is super important in many areas, but it’s making a big difference in healthcare. Data analytics helps improve healthcare in big ways, leading to discoveries and better ways to take care of people. A report by Grand View Research said that the healthcare data analytics market was worth a huge $26 billion in 2019 and it’s growing fast – expected to increase at an annual growth rate of 7.5% from 2020 to 2027.
This paradigm shift towards data-driven healthcare paves the way for smarter, more responsive, and more effective healthcare systems. It signifies a leap into a future where healthcare is not just reactive but predictive, not just curative but preventive, and where every decision is underpinned by robust, real-time data insights.
What is Healthcare Analytics?
Healthcare analytics refers to the systematic use of data and statistical analysis to improve healthcare delivery, outcomes, and management.Healthcare analytics involves the collection, analysis, and interpretation of health data from various sources, including electronic health records (EHRs), billing systems, insurance, medical imaging, genomic sequencing, payor records, pharmaceutical research, and more. By utilizing healthcare analytics, organizations can effectively identify patterns, trends, and correlations in their data. This approach is crucial for improving patient care, increasing operational efficiency, and enhancing financial results.
Types of Healthcare Analytics
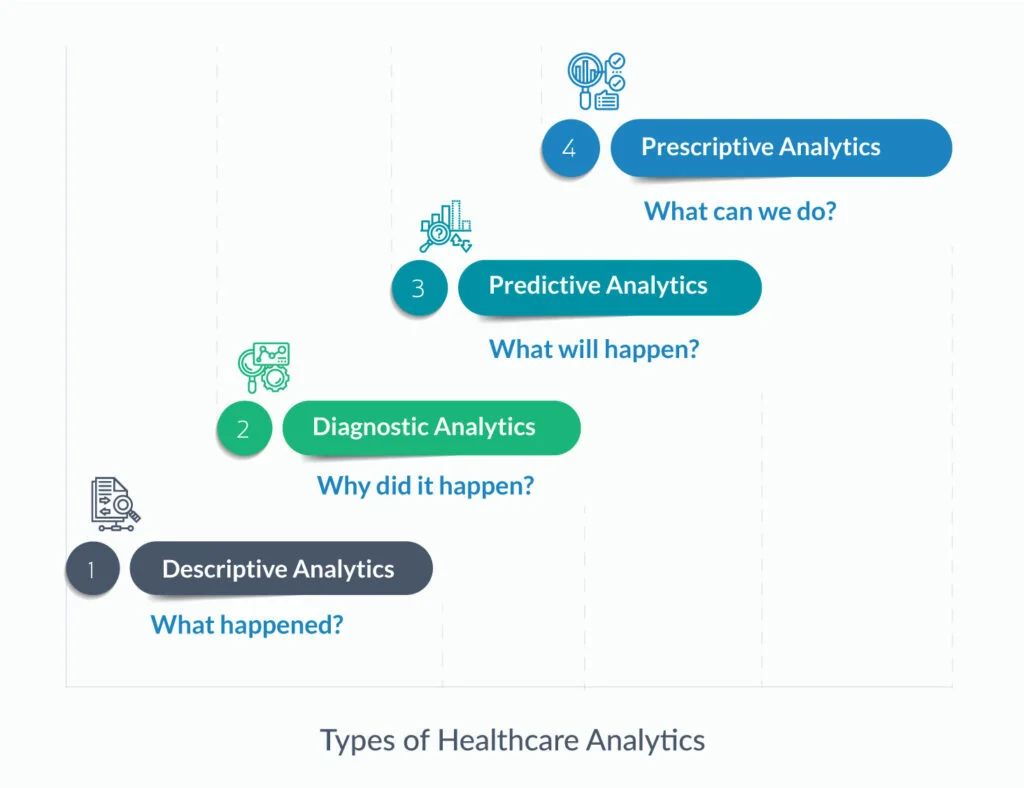
Descriptive Analytics
Descriptive analytics provides insights into what has happened historically and helps in answering questions like:
- How many patients were admitted to the hospital in the previous week?
- What proportion of patients discontinued home therapy in the past month?
- What are our patient population’s most common diagnoses among different age groups?
- What is the readmission rate for patients with chronic illnesses like diabetes or heart disease?
To obtain answers to these types of questions, historical data is analyzed using essential statistical tools such as counts, percentages, means, and standard deviations. Tracked over time, these metrics enable healthcare providers to assess whether their practices are achieving set objectives and where improvements might be necessary. It’s a foundational analytical method for understanding and improving healthcare outcomes.
Diagnostic Analytics
Diagnostic analytics goes a step further to explain why something happened and helps in answering questions like:
- Why did patients go to the hospital last week?
- Why has there been an increase in certain diseases or health conditions in a particular region?
- Why are certain patient groups experiencing higher readmission rates?
This analytical approach involves a more detailed exploration of data, often to interpret or elucidate findings obtained from descriptive analytics. For example, suppose a hospital notices through descriptive analytics that the readmission rates for heart failure patients are higher than the national average. Then, the next logical step is to determine why these readmissions are occurring. In diagnostic analytics, the hospital investigates various factors that could contribute to these high readmission rates. They analyze patient records to identify commonalities among readmitted patients, such as the severity of their initial condition, adherence to medication post-discharge, follow-up care level, and socio-economic factors like access to healthy food or a support system at home. The hospital might also explore whether there were any patterns in the discharge education provided to these patients or in the post-discharge support services.
By using statistical methods to examine these aspects, the hospital can better understand the factors leading to higher readmission rates. Just like in descriptive analytics, this process involves thoroughly investigating historical data to uncover the ‘why’ behind the observed trend.
Predictive Analytics
Predictive analytics is used to understand what will happen. It helps in predicting situations like:
- Which patients are at risk of being hospitalized in the coming week?
- Which individuals currently on home therapy will likely transition to in-centre treatments shortly?
- How many staff members will be needed in different hospital departments next month?
This type uses statistical models and forecasting techniques to understand the likelihood of future outcomes. These models can take advantage of thousands of data elements in the electronic record for each patient and identify patterns from all patients in the database. For example, a healthcare system can use algorithms to analyze electronic health records (EHRs) to identify patients at high risk of developing chronic diseases like diabetes. These patients can then be targeted for early intervention programs.
Prescriptive Analytics
This is the most advanced type of analytics which suggests actions to achieve desired outcomes. It allows us to understand what actions are needed to change the prediction, like in the following examples:
- For patients with chronic conditions, analytics could prescribe more frequent telehealth check-ins or adjust medication regimens based on real-time health data.
- In elderly patients with a high fall risk, prescriptive analytics might suggest home modifications, physical therapy regimens, and wearable devices to alert caregivers in case of a fall.
- Arranging a home visit from a social worker could prevent a patient from discontinuing home therapy.
- Prescriptive analytics can recommend the best ways to allocate limited medical resources like ventilators, ICU beds, and staff during a sudden influx of patients, such as in a pandemic.
By integrating insights from predictive analytics, prescriptive analytics not only identifies areas in need of improvement but also proposes concrete, data-driven solutions. This approach enhances decision-making, leading to superior patient outcomes and more efficient healthcare service delivery. Prescriptive analytics serves as a bridge between predictive insights and actionable healthcare strategies, embodying the most sophisticated level of data analysis in the healthcare sector.
Benefits of Healthcare Analytics for Providers and Payers
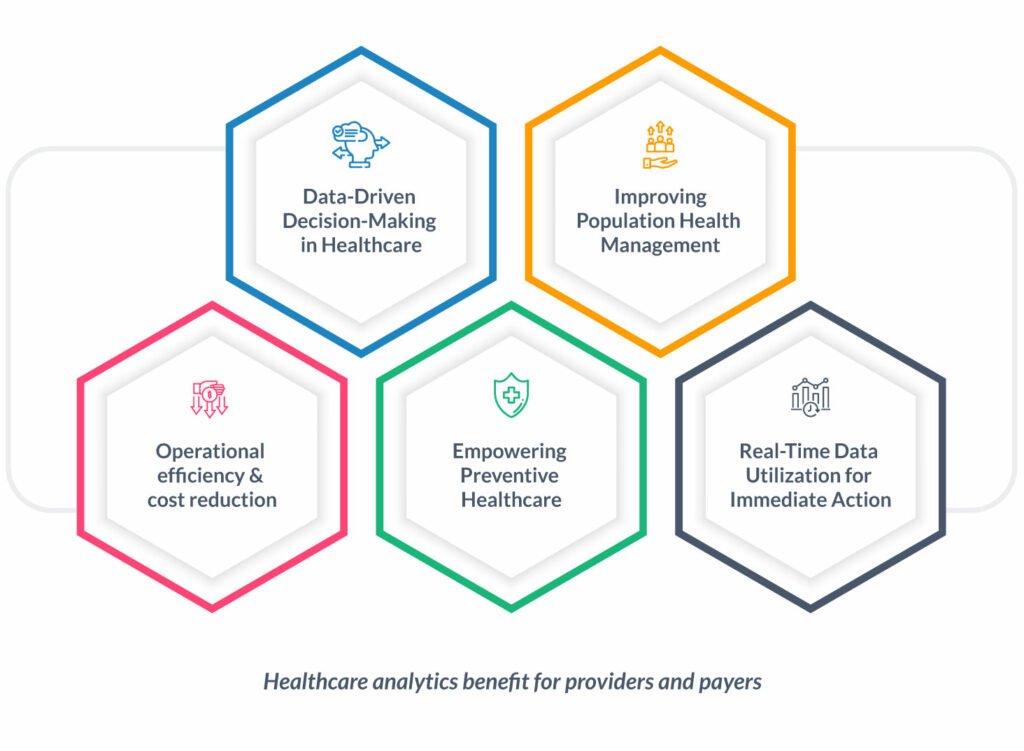
Data-Driven Decision-Making in Healthcare
Healthcare analytics plays a vital role in assisting decision-making. By integrating patient data, medical research, and evidence-based guidelines, healthcare professionals can access real-time insights to make informed treatment decisions. Clinical decision support systems leverage healthcare analytics to provide personalized recommendations, alert healthcare providers about potential adverse events, and improve patient outcomes.
Improving Population Health Management
A key benefit of healthcare analytics lies in its ability to provide a comprehensive perspective on population health. This holistic view enables healthcare entities to identify individuals at risk and craft useful intervention strategies. By meticulously analyzing data at a population level, trends in disease, public health concerns, and overall health patterns are more readily discernible. This intelligence is crucial in devising preventive care measures and health promotion strategies, ultimately fostering a healthier population.
Operational Efficiency and Cost Reduction
Healthcare analytics offers insights that can enhance operational efficiency and optimize costs. By analyzing data related to resource allocation, supply chain management, and financial planning, healthcare organizations can make data-driven decisions to improve workflows, reduce waste, and maximize the utilization of resources.
Empowering Preventive Healthcare
Analytics empowers patients with deeper insights into their health and treatment objectives, promoting preventive measures. This proactive approach in healthcare management encourages patients to engage more actively in their health journey, leading to better health outcomes.
Real-Time Data Utilization for Immediate Action
The real-time analysis of health data, right at the point of collection, enables healthcare providers to receive immediate alerts. This promptness ensures a swift response to patient needs and enhances the quality of care provided. By integrating real-time data analysis, healthcare systems can respond more dynamically to patient needs, enhancing overall healthcare delivery.
Real-World Applications of Healthcare Data Analytics
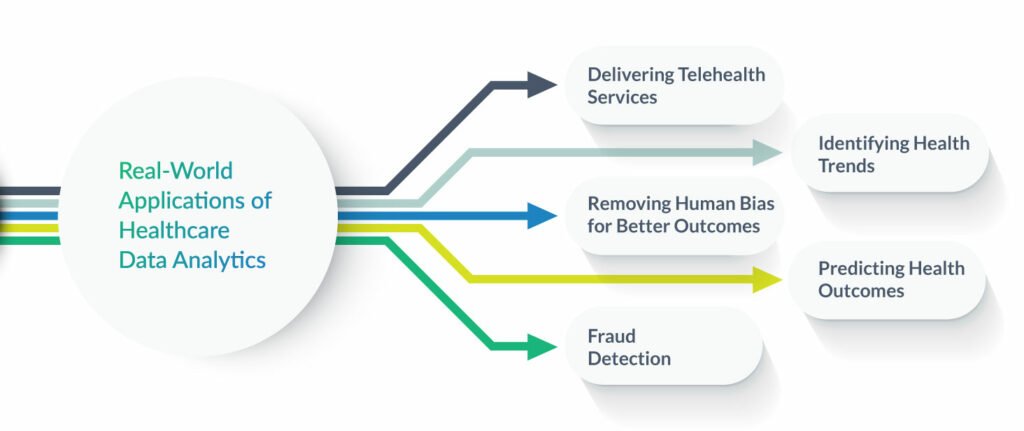
Delivering Telehealth Services
Healthcare data analytics significantly enhances telehealth services by enabling the collection and analysis of patient data remotely. This analysis helps in diagnosing and treating patients more effectively. For example, wearable devices can monitor vital signs in real time, and analytics can be used to detect anomalies or trends that require medical attention. This approach facilitates timely interventions and personalized care plans, improving patient outcomes. Data received from telehealth can be majorly helpful in predictive analysis as it provides real-time data of the users which makes providers make timely informed decisions.
Removing Human Bias for Better Outcomes
Healthcare data analytics can help in minimizing human biases in decision-making. By analyzing data patterns and patient outcomes, algorithms can provide evidence-based recommendations that are free from personal biases or prejudices. This objectivity can lead to more equitable healthcare delivery and improved patient outcomes, especially in areas like diagnosis, treatment options, and risk assessment. Descriptive data analytics can also be used to assess treatment outcomes across different patient groups to identify potential disparities or biases in care delivery.
Fraud Detection
Analytics plays a key role in detecting and preventing fraud in healthcare. By analyzing billing patterns, patient records, and insurance claims, data analytics tools identify irregularities that may indicate fraudulent activities. This not only saves costs for healthcare providers and insurers but also helps in maintaining integrity and trust in the healthcare system
Identifying Health Trends
Data analytics is pivotal in identifying broader health trends within populations. This includes tracking the spread of diseases, identifying risk factors for certain conditions, and understanding the effectiveness of public health interventions. By analyzing data using various descriptive, predictive, and diagnostic methods, healthcare professionals can make informed decisions about resource allocation and disease prevention strategies.
Predicting Health Outcomes
One of the most transformative applications of healthcare data analytics is in predicting patient outcomes. By leveraging historical data, current health data, and machine learning algorithms, healthcare providers can predict the likelihood of certain events, such as hospital readmissions, disease progression, or response to treatment. This predictive insight allows for proactive care and personalized treatment plans, potentially improving patient outcomes and reducing healthcare costs.
Where Would Healthcare Analytics Have The Most Impact?
The ability of data analytics to convert raw healthcare data into actionable intelligence is expected to have the greatest impact on these areas of healthcare:
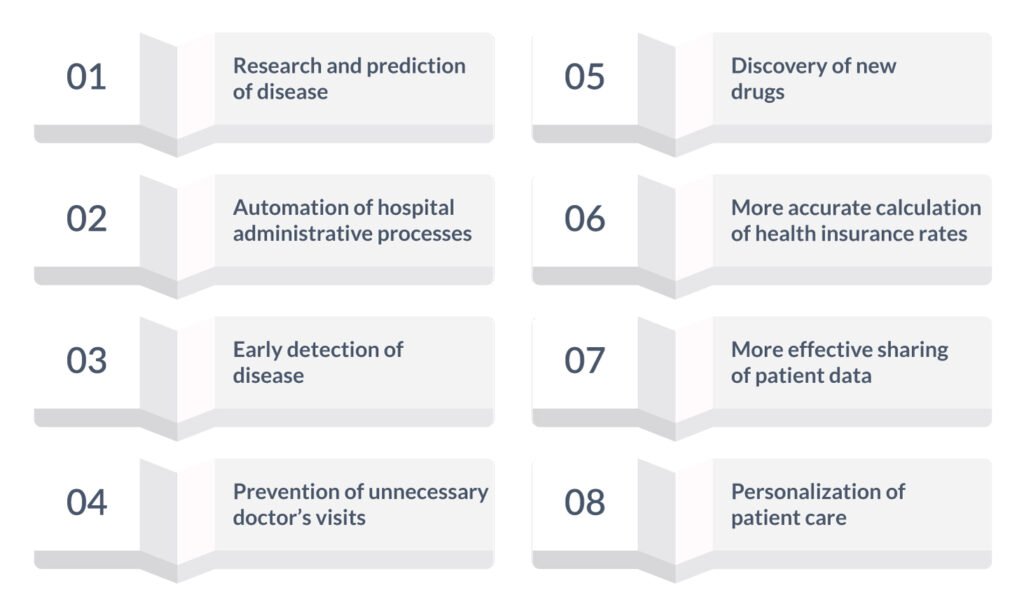
Tools Needed for Healthcare Data Analytics
The technological tools and software can be broadly classified into three distinct categories, each serving a pivotal role in the data management and analysis process:
Data Acquisition Software
This category encompasses a suite of tools designed for the collection and aggregation of data from a variety of sources. These sources range from direct patient feedback obtained through surveys to clinical data recorded in Electronic Health Records (EHRs), as well as real-time data streaming from wearable health devices. The primary function of these tools is to facilitate the seamless transfer and consolidation of diverse health data sets.
Interested to know how Digicorp can help you build a data acquisition tool? Get in touch with our team at sales@digicorp.com.
Data Processing Programs
Within this category are programs specifically engineered for the tasks of data cleansing, validation, and analysis. They are used for refining the raw data, ensuring its accuracy and reliability, and subsequently conducting detailed analysis. These programs are tailored to extract meaningful insights from the data with respect to specific research questions or investigative needs in the healthcare sector.
Analytical Software
These tools are designed to interpret the outcomes of the analysis and, in turn, generate actionable recommendations aimed at achieving specific healthcare objectives. They bridge the gap between data-driven insights and practical application, guiding healthcare providers toward targeted interventions and strategic decision-making for enhanced patient care and operational efficiency.
Our Expertise in the HealthTech Domain
With over 20 years of expertise in the healthtech sector, Digicorp stands at the forefront of innovative healthcare technology development. Our portfolio includes a diverse range of solutions, including Electronic Health Records (EHR) software, mHealth applications, digital therapeutics, and more.
How DIGICORP can assist you?
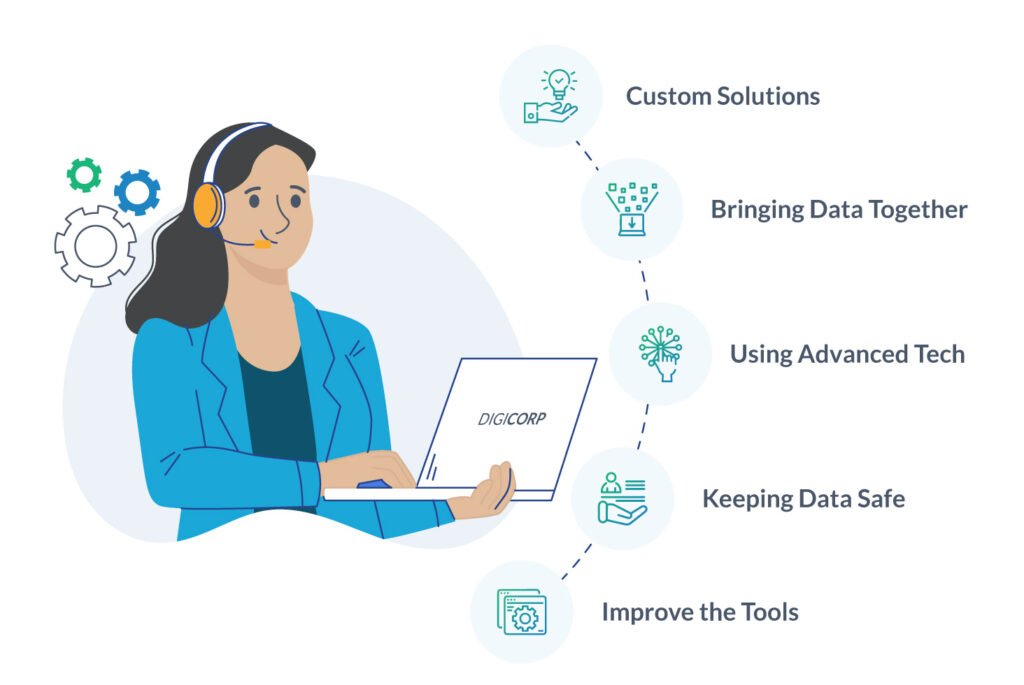
Custom Solutions: In creating specialized software that improves your clinical outcome and also in creating an advanced analytics tool to improve revenue cycle management.
Bringing Data Together: Combine different types of data, like patient records and info from wearable devices to get a complete picture of patient health and improve quality of care.
Using Advanced Tech: Employing smart tech like AI to predict health trends and suggest the best ways to take care of patients and improve decisions.
Keeping Data Safe: Secure patient information and adhere to HIPAA compliance.
Improve the Tools: Improve the ease of use of your platform and thereby reduce data entry time so that you can spend more time with patients.
In Conclusion
In today’s world, data holds the key to valuable insights, particularly in healthcare, where the paradigm shift towards data-driven decision-making is reshaping the industry. From descriptive to prescriptive analytics, the evolution sets the stage for a predictive, preventive, and deeply informed healthcare future. Benefits span data-driven decision-making, population health management, and operational efficiency, extending to real-world applications like telehealth services and fraud detection. Anticipated impacts include disease research, automation of administrative processes, and personalized patient care.
Crucial technological tools, such as data acquisition software and analytical programs, are driving this transformation. Companies like Digicorp provide expertise in healthtech for customized solutions, data integration, and enhanced efficiency. In summary, healthcare analytics signifies a transformative shift towards informed decisions, proactive care, and improved patient outcomes.
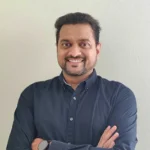
Sanket Patel
- Posted on February 6, 2024
Table of Contents